Sport is about the joy of the game. In hockey that means the sound of a skate scratching ice, the awe of a pass that unbelievably connects from one stick to another, or the thrill of a last second goal. But how those events come to mean something always brings us back to numbers. At the most important level, that's the scoreboard. Whichever team puts more pucks in the net wins the game. But beyond that, there is a wealth of information being recorded, measured, and calculated that dig into what is happening and help us mathematically predict what might happen next. These data points that go beyond a traditional scoresheet are called analytics, and if you are looking to understand them a little bit more, this story is for you!
Beyond the Box Score - An Intro to Hockey Analytics
Understanding the numbers that go deeper into the game of hockey: what they are, what they mean, and how to use them
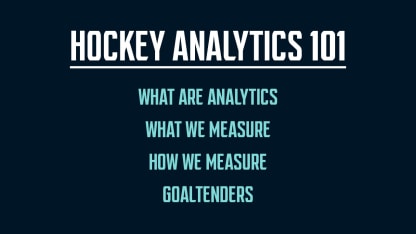
WHAT are Analytics?
The most important thing to understand about "analytics" is that unlike numbers that describe what happened (how many blocked shots in the game? How tall is a player? What is a team's win-loss record on a Tuesday?), analytics undergo mathematical rigor to prove that they have some meaningful predictive value. Information that is classified as "analytics" gives us a level of probability attached - if Team X does this, it's likely that this other thing happens. It's not guaranteed! But likely. Analytical-based numbers help us gain a deeper understanding of what a team or player really is, beyond just looking at results.
WHERE do Analytics come from?
It's easy to think that "analytics" are crazy mathematical formulas that may feel intimidating to understand, but that's not necessarily the case! In hockey, almost all publicly available data is based on data that the NHL keeps track of and publishes. In fact, the roots of hockey analytics are based solely in "shot data": where was a shot taken, by whom, what kind of shot was it, and what was the outcome? From there, some measures do bring in mathematical complexities, but from the start, hockey analytics has really been about what numbers do we have that truly hold meaning in understanding the game.
There are limitations to this data, too. There's a lot we don't currently have: where is the puck at any given time, where exactly are players in relation to a play. Should you dig even deeper into analytics, you will rightly find that today, there are questions we have that analytics can't answer because we don't have the information (hopefully, future player and puck tracking data will help. This is also why using video with data is key.) But for now, let's look at what analytical information is most commonly used in hockey and what it tells us.
WHAT WE MEASURE:
CORSI
Also Known As: Shot Attempts | Shots | Shot Volume | Possession
When you see a "shot count" on a scoreboard or a box score, that number represents only pucks that either go into the net (and become a goal) or are stopped by a goaltender. If you watch even a few minutes of hockey, you'll notice that a lot more pucks are directed towards the net than those that either go in or are played by a goalie. That's where Corsi comes in. Corsi doesn't just count shots in the traditional sense, it also counts missed shots (pucks that miss the net), and blocked shots. You can come up with this number on your own simply by adding the first three columns on an NHL scoresheet. Corsi can be measured "for" a team / player (CF) or "against" a team / player ("CA") as an on-ice measure or individual count.
Why is Corsi helpful? First, it's a more complete representation of the offense that a team is generating - it's all the pucks being sent towards the net - and it also represents the workload a goaltender faces.
Second, and most importantly, Corsi has been statistically proven to be one of the strongest predictors of the likelihood to win a game: the team that shoots the most pucks towards the net is most likely to get the ideal outcome! Next time you hear "the team tilted the ice in their favor," or "the team controlled possession," it's likely rooted in Corsi.
FENWICK
Also Known As: Unblocked Shot Attempts | Unblocked Shots | Unblocked Shot Volume
Now that we understand why Corsi is good, we can also understand where it has flaws. Currently, public shot data from the NHL is tracked by humans recording shot location and outcome, and this means, when it comes to blocked shots, the NHL marks where a shot is blocked not where it was shot from (there's only so much we can capture real time!) So, Fenwick is a measure that removes the data that doesn't truly represent what happened - it's Corsi without the blocked shots. In other words, Fenwick is shots on goal, goals, plus missed shots.
Fenwick isn't as statistically predictive as Corsi, but it does help us understand the differences in performance at a team or player level as it relates to blocked shots. Fenwick is also a big piece of more complex analytical measures so understanding what it is important.
EXPECTED GOALS
Also Known As: Shot Quality | xG
Expected goals is a measurement based on the idea that not all shots are created equal, and this makes sense, no? It would seem far more likely that a goal comes from a puck shot from in close to the net as compared to a shot that was fired from far away at the blue line, right?
That's the adjustment expected goal calculations try to answer. Using a mathematical model that factors in which kinds of shots become goals, expected goals factors in a variety of factors including, but not limited to: shot distance, shot type, time since last shot, game state (even strength versus power play or penalty kill), and shooter.
While expected goals feels like a great measure, it's still not necessarily the best one we have because of a few key reasons. First, not all expected goals are created equal. Every model has its own formula so it's important to understand what each does and does not include. Secondarily, because these models are based on publicly available data, some pieces of information are assumptions - for example, we don't know for sure if a shot is a rebound so we decide that if two shots happen in a certain location within a certain amount of time, it's a rebound.
Expected goals is a valuable tool, but always take the time to know which model you are using and what that model represents. A few to check out:
Evolving Hockey
;
MoneyPuck
;
HockeyViz
; Natural Stat Trick.
WINS ABOVE REPLACEMENT / GOALS ABOVE REPLACEMENT
Also Known As: WAR / GAR
If you are a fan of baseball, you've likely heard the terms WAR or "wins above replacement." WAR is a measure that looks at a lot of different data points to try and distill a player's value into one single number and that is how many "wins" does a player add (or subtract!) to their team as compared to a "replacement level" player. A replacement level player is a conceptual baseline of a player who neither adds nor subtracts value - their contribution is zero. Goals above replacement does the same thing as WAR, but looks at how many goals a player contributes. GAR can also be broken out into sub measures including offensive GAR, defensive GAR, etc. Just like expected goals, there are a few WAR and GAR models, and just like expected goals, the data we have access to today limits how robust these models can be.
Given the complexity of the game of hockey, it's certainly fair to question the validity of a "one number captures all" measure. Think of WAR and GAR as good places to start understanding a player's contribution that can point you towards the types of follow up questions you may have about how to truly evaluate that player.
MICRO STATS
Also Known As: Passing Data | Zone Entries / Exits | Player Tracking
Everything we've talked about to this point has been "shot-based," but the next exciting batch of data we can explore looks at things that are happening leading up to a shot. This information is currently lumped into a catch-all category called "micro stats" and falls into a few categories:
Passing data: Who is making passes on a team? Where is the pass? What is the outcome of the pass?
Transition data (zone exits and entries): How does a team get out of their own zone / into their offensive zone? Who makes this happen? Who tries to keep it from happening on the other team? How often do they do it? What is the outcome?
We are just at the beginning stages of understanding the true value of this kind of information, but we are already learning what kind of passes are most "dangerous" (most likely to lead to a goal), and what are the best ways to get the puck into the offensive zone. The only drawback to this data is that today, it is not made publicly available by the league and must be manually tracked. This means that getting our hands on this information is a much slower process than working with shot-based data.
HOW WE MEASURE:
What's great about "analytical" measurements is that just like more traditional stats, they can apply to a player or a team. But because analytical measures have predictive value, it's also important to understand the different ways these numbers can be applied to a situation.
Every measure can be totaled all together to give a count. For example, Phillip Grubauer had 100 saves. That tells us exactly how many pucks Grubauer stopped. But how does that stack up against other goaltenders? Or other seasons? No two players at any position play the exact same amount of time. How can we be sure we are comparing apples to apples? We address these kinds of questions by using different units of measure.
RATES
Also known as: Per 60 | / 60 | Per 60 minutes of play
If Grubauer plays 240 minutes across four games and stops 48 pucks is that better or worse than a goaltender who plays 594 minutes across 10 games and stops 100? If we just compared counts - 48 saves versus 100 - we would think that the player with 100 saves is "better." But that's not necessarily the case.
By rating stats - or accounting for how much time a player had to put forth the measured performance - we bring everyone onto the same scale to see how they did. The most common rate scale in hockey is "per 60 minutes of play" since that is how long a hockey game lasts. If we rate out the two examples above (# of pucks saved / total time played x 60) we find that Grubauer was actually saving 12 pucks per 60 minutes of play as compared to the other player who was saving 10 pucks per 60 minutes of play. Now which player is better?
By rating stats, we don't have to worry as much about variance in playing time. We can bring every player onto a common unit of measure. Of course, reasons for variance are always worth investigating - is a player injured? Playing protected minutes? Those are deeper questions. But at least with rates (which can be applied to all of the stats we've already discussed), we can remove some of the inequities of variance in playing time.
PERCENTAGES
Rates allow us to see how a player or team produces, but is that production enough? That's where percentages come in. Percentages allow us to look not just at production in an apples to apples way, but also to factor in how that production measures up when you consider what your competition is doing.
Let's look at another example. Let's say that we know that the Kraken produce 34 shot attempts per 60 and they are about to play a team that produces 42 shot attempts per 60. If this is all we looked at, it would be easy to assume that the other team created more offense, or, another thought might be that the other team was "better."
But what if we knew that while the Kraken take about 34 shot attempts per 60, the average number of shot attempts in any Kraken game by both team is 65. That means that the Kraken earn 52-percent of all shot attempts in any given game (34 divided by 65), and would be noted as a Corsi for percentage (CF%) or 52. Now, consider the other team. They take 42 shot attempts, but their games average 93 shot attempts by both teams. That means the other team earns about 45-percent of all shot attempts. So, while the Kraken have a lower overall shot count, they are playing in a way that they gain the advantage over their opponents.
THRESHOLDS
There's one important note to any measure, and that is from a mathematical perspective, we need a certain amount of data to ensure that it's truly representative of what might happen. For example, if a goaltender plays 20 minutes of a game and stops all 10 pucks they saw, they have a save percentage of 100%. Does that mean we assume that every time this goalie plays they will stop every puck sent their way? Of course not. Always take time to ensure you're looking at enough data to trust the meaning we give it. For most hockey stats, that's anywhere between 20-25 games. That can also be translated to minutes played.
WHEN WE MEASURE
Now we understand the kinds of numbers we look at and how they are measured, but there's one more variable that we have to consider, and that's game state. Most of a hockey game (hopefully!) is played with five skaters plus one goalie on the ice for each team. But, sometimes, due to penalties, one team has to play a skater down (penalty kill) while the other team has an extra player on the ice (power play). It makes sense to acknowledge that teams play differently if they have a different number of skaters on the ice and that's where game state comes in.
Game state groups all the kinds of data we've already reviewed by how many skaters are on the ice so what happens during a power play doesn't inflate or detract from how a team or player players the majority of the time which is with five skaters versus five skaters.
The groupings of game state that you might see include:
If you want to truly evaluate a player or team, it is best to look at even strength play not only because this represents the majority of the scenarios in which a player will play, but also, because there is so much five-on-five play, we have the largest amount of this data making it the most sound mathematically. Always make sure you know what game state(s) you are looking at when it comes to working with analytical data.
WHAT ABOUT GOALTENDERS?
We've talked a lot about the skaters on the ice, but not so much about goaltending. Play in net is arguably one of the least measured elements in hockey analytics, presently, and that's again in part because it's so hard to get reliable data quickly on what a goaltender is doing. We don't know for sure the angle of a shot, how was the goalie set up, or what a goalie does or does not see.
Those short-comings aside, we have some basic measures that help us better understand a goaltender. Just like we can measure shot quality skaters produce (or prevent) with expected goals, we can use xG to consider what kind of shot quality did a goaltender face? And just like we have replacement level skater values, we have replacement level (league average) goaltending that we can include in considering how a goaltender performs. Let's say that Grubauer allows two goals in a game. Is that good or bad? What if the expected goals against (xGA) was 5.2? Grubauer saw the kinds of shots that should have resulted in 5.2 goals against but he only let in two pucks, stopping over three goals against! That's pretty good. That measure is called "Goals Saved Above Expectations" or GSAx.
Similarly, we can look at a goaltenders expected save percentage against all unblocked shots (xFSV%) and see if they were above or below that number - what was the differential? (dFSV%).
Any goaltending analyst will tell you these numbers still don't fully capture goaltending performance, and they are likely right. But for now, with the public data we have, these are our starting grounds.
WHAT'S MISSING?
As we said at the start, there's still so much to explore in hockey analytics, and today, public work has only shot data to work with unless manually tracked data is added in. Other sports like professional soccer, NBA basketball, NFL football and MLB baseball are a bit further down the road both in terms of the data they've been able to work with and also the studies they've been able to complete. If you start diving into analytics, you will likely come across questions that you still can't truly answer or answers that seem incomplete. That is OK! It's these questions that will help drive future evolutions in what numbers can help us understand the game.
Additional Hockey Analytics 101 Articles
READ:
Why We Use Game States